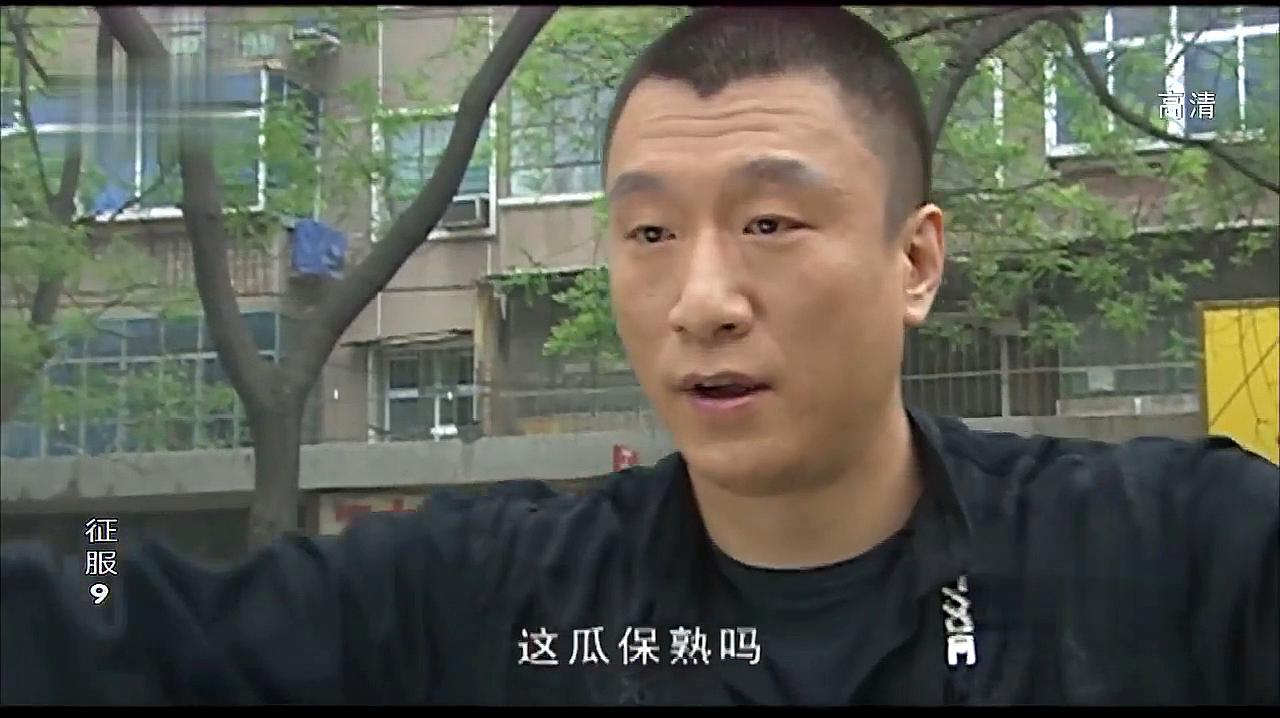
在机器学习领域,有一个很有名气的西瓜--周志华老师的《机器学习》,很多同学选择这本书入门,都曾有`被西瓜支配的恐惧`。我写文章的时候也特别喜欢用西瓜数据集,以它为例手算+可视化讲解过XGBoost,自认非常通俗易懂。
最近我介绍了决策树的可视化,还有可以快速实现机器学习web应用的神器——streamlit 今天我们就把它们结合起来,用机器学习帮华强挑西瓜!仅供娱乐,希望大家可以学到一些新姿势。
> 项目已发布,欢迎大家试玩
> https://share.streamlit.io/tjxj/watermelon-prediction/main/app.py
老规矩,先看效果图(GIF刷新有点慢,请耐心等待)
%20(1)_20211213194519.gif)
%20(3)_20211213194519.gif)
## 使用方法
第一步,左侧先选择西瓜外观
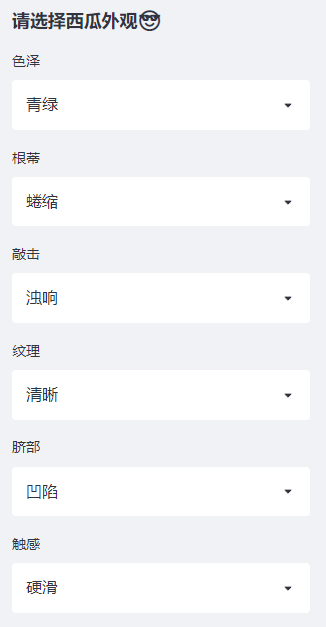
第二步,选择决策树的模型参数
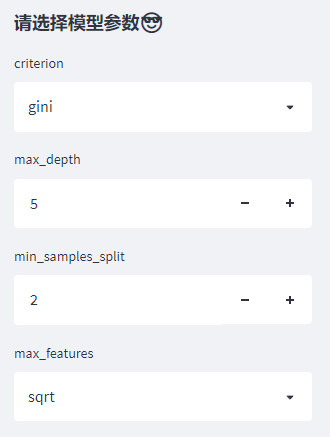
第三步,看结果
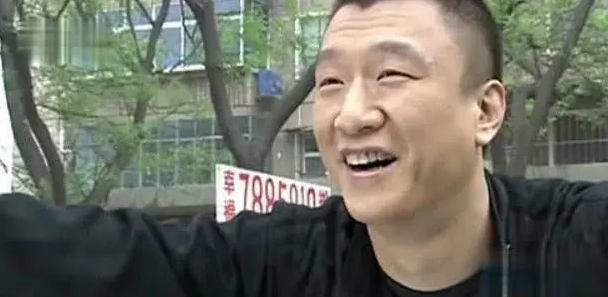
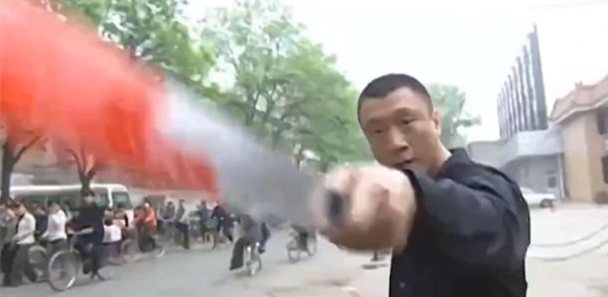
## 实现方式
`注:`篇幅原因,仅贴出核心代码

### data.py
主要是原始数据的处理,inputData方法实现输入外观变量值的标签编码。
```
def inputData():
st.sidebar.subheader("请选择西瓜外观:sunglasses:")
color = st.sidebar.selectbox("色泽", ("青绿", "乌黑", "浅白"))
root = st.sidebar.selectbox("根蒂", ("蜷缩", "稍蜷", "硬挺"))
knocks = st.sidebar.selectbox("敲击", ("浊响", "沉闷", "清脆"))
texture = st.sidebar.selectbox("纹理", ("清晰", "稍糊", "模糊"))
navel = st.sidebar.selectbox("脐部", ("凹陷", "稍凹", "平坦"))
touch = st.sidebar.selectbox("触感", ("硬滑", "软粘"))
input = [[color, root, knocks, texture, navel, touch]]
features = ["color", "root", "knocks", "texture", "navel", "touch"]
np.array(input).reshape(1, 6)
df_input = pd.Dataframe(input, columns=features, index=None)
for feature in features[0:6]:
le = joblib.load("./models/" + feature + "_LabelEncoder.model")
df_input[feature] = le.transform(df_input[feature])
return df_input
```
### 训练模型及预测
这一块很简单,就不多解释了。注:数据量太小就不整交叉验证了
```
def dt_param_selector():
st.sidebar.subheader("请选择模型参数:sunglasses:")
criterion = st.sidebar.selectbox("criterion", ["gini", "entropy"])
max_depth = st.sidebar.number_input("max_depth", 1, 50, 5, 1)
min_samples_split = st.sidebar.number_input(
"min_samples_split", 1, 20, 2, 1)
max_features = st.sidebar.selectbox(
"max_features", [None, "auto", "sqrt", "log2"])
params = {
"criterion": criterion,
"max_depth": max_depth,
"min_samples_split": min_samples_split,
"max_features": max_features,
}
model = DecisionTreeClassifier(**params)
df = dataPreprocessing()
X, y = df[df.columns[:-1]], df["label"]
model.fit(X, y)
return model
```
```
def predictor():
df_input = inputData()
model = dt_param_selector()
y_pred = model.predict(df_input)
if y_pred == 1:
goodwatermelon = Image.open("./pics/good.png")
st.image(goodwatermelon,width=705,use_column_width= True)
st.markdown("
else:
file_ = open("./pics/bad2.gif", "rb")
contents = file_.read()
data_url = base64.b64encode(contents).decode("utf-8")
file_.close()
st.markdown(
f'',
unsafe_allow_html=True,
)
st.markdown('
return y_pred,model
```
### 决策树可视化
决策树可视化和插入网页我用`decisionTreeViz`和``svg_write``实现,可惜目前仅本地模式正常,发布后报错,尚未解决。
```
def decisionTreeViz():
df,le = getDataSet()
X, y = df[df.columns[:-1]], df["label"]
clf = joblib.load('..watermelonClassifier.pkl')
viz = dtreeviz(clf,
X,
y,
orientation="LR",
target_name='label',
feature_names=df.columns[:-1],
class_names=["good","bad"]
)
return viz
def svg_write(svg, center=True):
"""
Disable center to left-margin align like other objects.
"""
# Encode as base 64
b64 = base64.b64encode(svg.encode("utf-8")).decode("utf-8")
# Add some CSS on top
css_justify = "center" if center else "left"
css = f'
'
html = f'{css}'
# Write the HTML
st.write(html, unsafe_allow_html=True)
```
### streamlit
过程就不说了,就把调用的streamlit API列一下吧
```
st.title
st.write
st.code
st.table
st.markdown
st.graphviz_chart
st.sidebar
st.expander
st.code
st.image
st.pyplot
```
以上API具体用法大家可以查一查
`https://docs.streamlit.io/library/api-reference`
## TODO
- 增加更多模型
- dtreeviz决策树可视化bug
决策树可视化依赖graphviz,在localhost:8501下预览可以显示,发布到streamlit.io就不行了。
- 图片和GIF 不适配手机屏幕
参考:
https://mp.weixin.qq.com/s/QJe8BLXjyDtxA8PdtnkB0Q
欢迎分享,转载请注明来源:内存溢出
评论列表(0条)