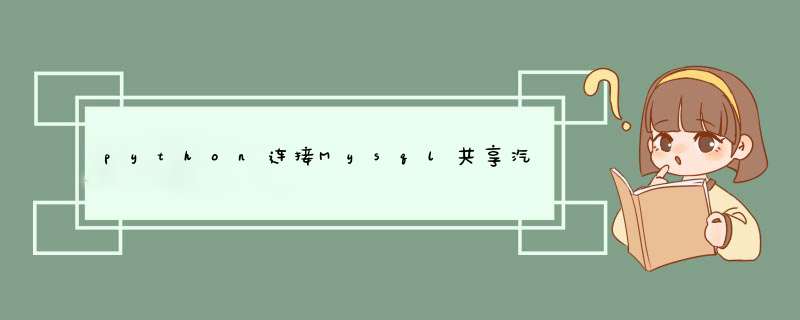
概述学习记录——
共享汽车分析前言本文仅记录个人学习过程写的代码供自己复盘使用,如果对你有帮助和启发那就更好了,新人作品,欢迎讨论和斧正,大神轻喷。纯代码实现,无结论。一些相似的维度举一反三就行。需要数据集练习的可以留言目标python,mysql,matplotlib代码练习常用数据 学习记录——共享汽车分析前言本文仅记录个人学习过程写的代码供自己复盘使用,如果对你有帮助和启发那就更好了,新人作品,欢迎讨论和斧正,大神轻喷。纯代码实现,无结论。一些相似的维度举一反三就行。需要数据集练习的可以留言目标python, MysqL, matplotlib 代码练习常用数据指标的实现数据导入
python 连接数据库 MysqL
#获取数据方法import pyMysqL import pandas as pd from matplotlib import pyplot as pltfrom matplotlib import Font_managerdef get_MysqL_data(DB, sql): conn = pyMysqL.connect(host=DB['host'], port=DB['port'], user=DB['user'], password=DB['password'], database=DB['dbname']) # 创建游标 cursor = conn.cursor() # 执行SQL语句 cursor.execute(sql) # 调出数据 data = cursor.fetchall() # cols为字段信息 cols = cursor.description # 将数据转换为DataFrame col = [] for i in cols: col.append(i[0]) # data转成List形式 data = List(map(List, data)) data = pd.DataFrame(data,columns=col) # 关闭游标以及连接 cursor.close() conn.close() return data
日期时间处理——获取年月日时维度
def time_data(data): data['下单年'] = data["下单时间"].astype("str").apply(lambda x: x.split('-')[0]) data['下单年月'] = data["下单时间"].astype("str").apply(lambda x: x.split('-')[0]+"/"+x.split('-')[1]) data['下单月'] = data["下单时间"].astype("str").apply(lambda x: x.split('-')[1]) data['下单日'] = data["下单时间"].astype("str").apply(lambda x: x.split('-')[2]) data['下单时'] = data["下单时间"].astype("str").apply(lambda x: x.split('-')[0]) data['付款年'] = data["付款日期"].astype("str").apply(lambda x: x.split('-')[0]) data['付款年月'] = data["付款日期"].astype("str").apply(lambda x: x.split('-')[0]+"/"+x.split('-')[1]) data['付款月'] =data["付款日期"].astype("str").apply(lambda x: x.split('-')[1]) data['付款日'] = data["付款日期"].astype("str").apply(lambda x: x.split('-')[2]) data['付款时'] = data["付款日期"].astype("str").apply(lambda x: x.split('-')[0]) return dataDB = { 'host':"127.0.0.1",'port':3306, 'user':'root','password':'password','dbname':'datebase'}
留存分析
# 留存分析sql='''select *,concat(ROUND(100*次日留存用户数/日活跃用户数),"%") 次日留存率,concat(ROUND(100*三日留存用户数/日活跃用户数),"%") 三日留存率,concat(ROUND(100*七日留存用户数/日活跃用户数),"%") 七日留存率from(select date(a.`付款时间`) 日期 ,DATE_FORMAT(a.`付款时间`,"%H") 时间,COUNT(disTINCT a.`用户ID`) 日活跃用户数,COUNT(disTINCT b.`用户ID`) 次日留存用户数,COUNT(disTINCT c.`用户ID`) 三日留存用户数,COUNT(disTINCT d.`用户ID`) 七日留存用户数from paper_data a left join paper_data b on a.`用户ID`=b.`用户ID`and date(b.`付款时间`)=date(a.`付款时间`)+1left join paper_data con a.`用户ID`=c.`用户ID`and date(c.`付款时间`)=date(a.`付款时间`)+3left join paper_data don a.`用户ID`=d.`用户ID`and date(d.`付款时间`)=date(a.`付款时间`)+7where a.`付款时间` between "2020-01-01" and "2020-01-31"GROUP BY date(a.`付款时间`))f;'''liuchun = get_MysqL_data(DB, sql)liuchunx=liuchun['日期']y1=liuchun['次日留存率']y2=liuchun['三日留存率']y3=liuchun['七日留存率']plt.figure(figsize=(25,10),dpi=80)my_Font = Font_manager.FontPropertIEs(fname="C:/Users/wty_pc/Anaconda3/lib/site-packages/matplotlib/mpl-data/Fonts/ttf/STSONG.TTF",size = 18)rects1=plt.plot(x,y1,color="red",Alpha=0.5,line,linewidth=3,label="次日留存率")rects2=plt.plot(x,y2,color="g",Alpha=0.5,line,linewidth=3,label="三日留存率")rects3=plt.plot(x,y3,color="b",Alpha=0.5,line,linewidth=3,label="七日留存率")rects4=plt.legend(prop=my_Font,loc="best")for i in range(len(x)): plt.text(x[i],y1[i],y1[i],Fontsize=15,ha="center")for i in range(len(x)): plt.text(x[i],y2[i],y2[i],Fontsize=15,ha="center")for i in range(len(x)): plt.text(x[i],y3[i],y3[i],Fontsize=15,ha="center") plt.show()
订单分析
订单分析(可以把订单换成其他的,比如用户数,销售量等数据)
# 1.根据下单的时间来统计订单量# (1)年维度sql='''select date_format(付款时间,"%Y")下单年 ,count(订单ID) as 订单量 from paper_data group by date_format(付款时间,"%Y")'''order_num_year=get_MysqL_data(DB, sql)my_Font = Font_manager.FontPropertIEs(fname="C:/Users/wty_pc/Anaconda3/lib/site-packages/matplotlib/mpl-data/Fonts/ttf/STSONG.TTF",size = 18)x=order_num_year["下单年"]y=order_num_year["订单量"]plt.figure(figsize=(20,8),dpi=80)rects = plt.bar(x,y,wIDth=0.3,color="b",label="2019")# 标注for rect in rects: height=rect.get_height() plt.text(rect.get_x()+rect.get_wIDth()/2,height+0.3,str(height),ha="center")plt.Title("年度订单量",FontPropertIEs=my_Font)plt.xlabel("年份",FontPropertIEs=my_Font)plt.ylabel("订单量",FontPropertIEs=my_Font)plt.legend(prop=my_Font,loc="best")plt.show()
# 订单分析# 1.根据下单的时间来统计订单量# (2)年月维度sql='''select date_format(付款时间,"%Y/%m")下单年月 ,count(订单ID) as 订单量 from paper_data group by date_format(付款时间,"%Y/%m")'''order_num_month=get_MysqL_data(DB, sql)my_Font = Font_manager.FontPropertIEs(fname="C:/Users/wty_pc/Anaconda3/lib/site-packages/matplotlib/mpl-data/Fonts/ttf/STSONG.TTF",size = 18)x=order_num_month["下单年月"]y=order_num_month["订单量"]plt.figure(figsize=(20,8),dpi=80)rects = plt.bar(x,y,wIDth=0.3,color=["r","g","b"])# 标注for rect in rects: height=rect.get_height() plt.text(rect.get_x()+rect.get_wIDth()/2,height+0.3,str(height),ha="center")plt.show()
# (3)年月、城市维度(折线图)sql='''select date_format(下单时间,"%Y/%m")下单年月 ,城市,count(订单ID) as 订单量 from paper_datagroup by date_format(下单时间,"%Y/%m"),城市order by date_format(下单时间,"%Y/%m");'''order_num_month1=get_MysqL_data(DB, sql)#把每个城市的数据取出来order_month_city=order_num_month1.groupby(["城市"])order_shanghai=order_month_city.get_group("上海").reset_index(drop="true")order_beijing=order_month_city.get_group("北京").reset_index(drop="true")order_hangzhou=order_month_city.get_group("杭州").reset_index(drop="true")order_xian=order_month_city.get_group("西安").reset_index(drop="true")# matplotlib画图部分my_Font = Font_manager.FontPropertIEs(fname="C:/Users/wty_pc/Anaconda3/lib/site-packages/matplotlib/mpl-data/Fonts/ttf/STSONG.TTF",size = 18)plt.figure(figsize=(25,10),dpi=80)plt.plot(order_shanghai["下单年月"],order_shanghai["订单量"],marker='o',color="r",label="上海订单量")plt.plot(order_beijing["下单年月"],order_beijing["订单量"],marker='v',color="g",label="北京订单量")plt.plot(order_hangzhou["下单年月"],order_hangzhou["订单量"],marker='^',color="b",label="杭州订单量")plt.plot(order_xian["下单年月"],order_xian["订单量"],marker='.',color="y",label="西安订单量")plt.xlabel("时间",FontPropertIEs=my_Font)plt.ylabel("订单量",FontPropertIEs=my_Font)plt.Title("各城市每月订单量",FontPropertIEs=my_Font)plt.legend(prop=my_Font,loc="best")# df.IDxmax()默认值是0,求列最大值的行索引,1就是反过来def city_max(df): return df.IDxmax()# 最大值标记plt.text(order_shanghai["下单年月"].iloc[city_max(order_shanghai["订单量"])],order_shanghai.max()["订单量"]+5,"上海最大订单:{}".format(order_shanghai.max()["订单量"]),Fontsize=12,ha="center",color="r",FontPropertIEs=my_Font)plt.text(order_beijing["下单年月"].iloc[city_max(order_beijing["订单量"])],order_beijing.max()["订单量"]+5,"北京最大订单:{}".format(order_beijing.max()["订单量"]),Fontsize=12,ha="center",color="g",FontPropertIEs=my_Font)plt.text(order_hangzhou["下单年月"].iloc[city_max(order_hangzhou["订单量"])],order_hangzhou.max()["订单量"]+5,"杭州最大订单:{}".format(order_hangzhou.max()["订单量"]),Fontsize=12,ha="center",color="b",FontPropertIEs=my_Font)plt.text(order_xian["下单年月"].iloc[city_max(order_xian["订单量"])],order_xian.max()["订单量"]+5,"西安最大订单:{}".format(order_xian.max()["订单量"]),Fontsize=12,ha="center",color="y",FontPropertIEs=my_Font)plt.show()# -----------------------------# 每年、每月的一样的做法,改下日期字段就好
# (3)年城市维度(柱状图)sql='''select date_format(付款时间,"%Y")下单年,城市,count(订单ID) as 订单量 from paper_datagroup by date_format(付款时间,"%Y"),城市order by date_format(付款时间,"%Y");'''order_num_year=get_MysqL_data(DB, sql)# order_num_month.describe()# 按城市拆分数据order_year_city=order_num_year.groupby(["城市"])order_shanghai=order_year_city.get_group("上海").reset_index(drop="true")order_beijing=order_year_city.get_group("北京").reset_index(drop="true")order_hangzhou=order_year_city.get_group("杭州").reset_index(drop="true")order_xian=order_year_city.get_group("西安").reset_index(drop="true")# -----matplotlib画图部分------my_Font = Font_manager.FontPropertIEs(fname="C:/Users/wty_pc/Anaconda3/lib/site-packages/matplotlib/mpl-data/Fonts/ttf/STSONG.TTF",size = 18)plt.figure(figsize=(25,10),dpi=80)# 设置柱子位置,通过偏移量a来实现,# 由于时间是datetime类型或者object(str)类型的不能直接加偏移量所以这里用长度长度实现柱子的位置,然后把刻度值重新设置 a=0.2 x_01=List(range(len(order_shanghai["下单年"])))x_02=[i+a for i in x_01]x_03=[i-2*a for i in x_01]x_04=[i-a for i in x_01]# 画图rects1=plt.bar(x_01,order_shanghai["订单量"],a,color="r",align='edge',label="上海订单量")rects2=plt.bar(x_02,order_beijing["订单量"],a,color="g",align='edge',label="北京订单量")rects3=plt.bar(x_03,order_hangzhou["订单量"],a,color="b",align='edge',label="杭州订单量")rects4=plt.bar(x_04,order_xian["订单量"],a,color="y",align='edge',label="西安订单量")# x轴刻度plt.xticks(x_01,labels=order_shanghai["下单年"])# 轴名称plt.xlabel("年份",FontPropertIEs=my_Font)plt.ylabel("订单量",FontPropertIEs=my_Font)# 标题plt.Title("各城市每月订单量",FontPropertIEs=my_Font)# 图例plt.legend(prop=my_Font,loc="best")# 标记def mark_bar(rects): for rect in rects: height=rect.get_height() plt.text(rect.get_x()+rect.get_wIDth()/2,height+0.3,str(height),Fontsize=15,ha="center")mark_bar(rects1)mark_bar(rects2)mark_bar(rects3)mark_bar(rects4)plt.show()
# 3、小时订单量分布情况sql= '''SELECT date_format(下单时间,"%H") 下单时, count(`订单ID`) 订单量from paper_datawhere date_format(下单时间,"%Y-%m")="2020-01"GROUP BY date_format(下单时间,"%H") ORDER BY date_format(下单时间,"%H") ;'''h_dingdan=get_MysqL_data(DB, sql)my_Font = Font_manager.FontPropertIEs(fname="C:/Users/wty_pc/Anaconda3/lib/site-packages/matplotlib/mpl-data/Fonts/ttf/STSONG.TTF",size = 18)plt.figure(figsize=(25,10),dpi=80)x=h_dingdan["下单时"]y=h_dingdan["订单量"]plt.plot(x,y,color="r",label="每小时订单量")# 设置标记for a,b in zip(x,y): #a x坐标, b坐标, (a,b) 一个点 # ha 水平方向 va 垂直方向 Fontsize 大小 plt.text(a,b,b ,ha='center',va='bottom', Fontsize=12)#设置刻度xtick_labels = ['{}:00'.format(i) for i in x]plt.xticks(x,xtick_labels)#设置坐标名称plt.xlabel("时间",FontPropertIEs=my_Font)plt.ylabel("订单量",FontPropertIEs=my_Font)#设置表名plt.Title("1月每小时订单量",FontPropertIEs=my_Font)#设置图例plt.legend(prop=my_Font,loc="best")plt.show()
RFM模型
#RMF分层sql='''SELECT 用户ID,COUNT(订单ID) AS F,round(sum(实收金额),2) AS M,date_format(max(付款时间),"%Y/%m/%d") as R FROM paper_data GROUP BY 用户ID ORDER BY 用户ID ;'''User_layer=get_MysqL_data(DB, sql)# RMF评分公式# User_layer["R1"]=((pd.to_datetime("2020/03-01")-pd.to_datetime(User_layer["R"])) 后面的处理方式是为了把timedelta类型转换成Int类型User_layer["R1"]=((pd.to_datetime("2020/03-01")-pd.to_datetime(User_layer["R"]))/pd.timedelta(1,"D")).fillna(0).astype(int)User_layer["RR"]=(pd.to_datetime(User_layer["R"])-pd.to_datetime(min(User_layer["R"])))/((pd.to_datetime(max(User_layer["R"]))-pd.to_datetime(min(User_layer["R"]))))User_layer["FF"]=(User_layer["F"]-min(User_layer["F"]))/(max(User_layer["F"])-min(User_layer["F"]))User_layer["MM"]=(User_layer["M"]-min(User_layer["M"]))/(max(User_layer["M"])-min(User_layer["M"]))User_layer["score"]=100*(0.25*User_layer["FF"]+0.6*User_layer["MM"]+0.15*User_layer["RR"])User_layer
| 用户ID | F | M | R | R1 | RR | FF | MM | score |
---|
0 | 1 | 32 | 3517.0 | 2021/01/25 | -330 | 0.980519 | 0.673913 | 0.663953 | 71.392801 |
---|
1 | 10 | 29 | 2600.0 | 2021/01/23 | -328 | 0.977922 | 0.608696 | 0.490345 | 59.306896 |
---|
2 | 100 | 31 | 3906.0 | 2021/01/11 | -316 | 0.962338 | 0.652174 | 0.737599 | 74.995376 |
---|
3 | 1000 | 24 | 2255.0 | 2021/01/07 | -312 | 0.957143 | 0.500000 | 0.425028 | 52.358847 |
---|
4 | 10009 | 1 | 71.0 | 2019/04/09 | 327 | 0.127273 | 0.000000 | 0.011549 | 2.602010 |
---|
... | ... | ... | ... | ... | ... | ... | ... | ... | ... |
---|
5924 | 9977 | 1 | 19.0 | 2020/10/27 | -240 | 0.863636 | 0.000000 | 0.001704 | 13.056779 |
---|
5925 | 998 | 34 | 3634.0 | 2021/02/06 | -342 | 0.996104 | 0.717391 | 0.686104 | 74.042566 |
---|
5926 | 999 | 33 | 3356.0 | 2021/01/27 | -332 | 0.983117 | 0.695652 | 0.633472 | 70.146388 |
---|
5927 | 9993 | 1 | 125.0 | 2020/11/04 | -248 | 0.874026 | 0.000000 | 0.021772 | 14.416713 |
---|
5928 | 9994 | 1 | 135.0 | 2020/08/22 | -174 | 0.777922 | 0.000000 | 0.023665 | 13.088748 |
---|
5929 rows × 9 columns
# 根据权重score四分位分组的依据User_describe=User_layer.describe()User_describe
| F | M | R1 | RR | FF | MM | score |
---|
count | 5929.000000 | 5929.000000 | 5929.000000 | 5929.000000 | 5929.000000 | 5929.000000 | 5929.000000 |
---|
mean | 10.979086 | 1148.957160 | -117.514083 | 0.704564 | 0.216937 | 0.215630 | 28.929667 |
---|
std | 13.656880 | 1440.872815 | 228.595026 | 0.296877 | 0.296889 | 0.272789 | 26.743116 |
---|
min | 1.000000 | 10.000000 | -345.000000 | 0.000000 | 0.000000 | 0.000000 | 0.185039 |
---|
25% | 1.000000 | 112.000000 | -318.000000 | 0.489610 | 0.000000 | 0.019311 | 8.927758 |
---|
50% | 2.000000 | 198.000000 | -208.000000 | 0.822078 | 0.021739 | 0.035593 | 14.496745 |
---|
75% | 26.000000 | 2679.000000 | 48.000000 | 0.964935 | 0.543478 | 0.505301 | 58.371452 |
---|
max | 47.000000 | 5292.000000 | 425.000000 | 1.000000 | 1.000000 | 1.000000 | 97.960474 |
---|
# df遍历后的结果最好用List/dict存放起来。df本身一个一个添加数据遍历效率不高。而且难取数,最好用List/dict整体取赋值List_level=[]for index ,rows in User_layer.iterrows():# print("索引是:",index,"数据是:",rows) if rows["score"]<=User_describe.loc["25%"]["score"]: rows["level"]="四等" elif rows["score"]<=User_describe.loc["50%"]["score"]: rows["level"]="三等" elif rows["score"]<=User_describe.loc["75%"]["score"]: rows["level"]="二等" else: rows["level"]="一等" List_level.append(rows["level"]) User_layer['level']=List_levelUser_layer
| 用户ID | F | M | R | R1 | RR | FF | MM | score | level |
---|
0 | 1 | 32 | 3517.0 | 2021/01/25 | -330 | 0.980519 | 0.673913 | 0.663953 | 71.392801 | 一等 |
---|
1 | 10 | 29 | 2600.0 | 2021/01/23 | -328 | 0.977922 | 0.608696 | 0.490345 | 59.306896 | 一等 |
---|
2 | 100 | 31 | 3906.0 | 2021/01/11 | -316 | 0.962338 | 0.652174 | 0.737599 | 74.995376 | 一等 |
---|
3 | 1000 | 24 | 2255.0 | 2021/01/07 | -312 | 0.957143 | 0.500000 | 0.425028 | 52.358847 | 二等 |
---|
4 | 10009 | 1 | 71.0 | 2019/04/09 | 327 | 0.127273 | 0.000000 | 0.011549 | 2.602010 | 四等 |
---|
... | ... | ... | ... | ... | ... | ... | ... | ... | ... | ... |
---|
5924 | 9977 | 1 | 19.0 | 2020/10/27 | -240 | 0.863636 | 0.000000 | 0.001704 | 13.056779 | 三等 |
---|
5925 | 998 | 34 | 3634.0 | 2021/02/06 | -342 | 0.996104 | 0.717391 | 0.686104 | 74.042566 | 一等 |
---|
5926 | 999 | 33 | 3356.0 | 2021/01/27 | -332 | 0.983117 | 0.695652 | 0.633472 | 70.146388 | 一等 |
---|
5927 | 9993 | 1 | 125.0 | 2020/11/04 | -248 | 0.874026 | 0.000000 | 0.021772 | 14.416713 | 三等 |
---|
5928 | 9994 | 1 | 135.0 | 2020/08/22 | -174 | 0.777922 | 0.000000 | 0.023665 | 13.088748 | 三等 |
---|
5929 rows × 10 columns
用户标签
# 用户打标签sql='''select 用户ID,count(*) as order_num,count(date_format(付款时间,"%M")) 月数, sum(case when 取车类型='自取' then 1 else 0 end) as 自取次数, sum(case when 优惠金额>1 then 1 else 0 end) as 优惠券使用次数, sum(case when 非网点还车费>0 then 1 else 0 end) as 还车次数 from paper_data group by 用户ID ;'''bq=get_MysqL_data(DB, sql)data_total=pd.merge(User_layer,bq,on="用户ID")data_total# print(data_total["FF"].dtypes,data_total["自取次数"].dtypes)for index,row in data_total.iterrows():# 取车 if row["FF"]==0: row["FF"]= row["FF"]+0.0001# Int/浮点数会报错,注意转换类型 if float(row["自取次数"])/row["FF"]>0.5: data_total["取车偏好"]="时租居多" else: data_total["取车偏好"]="日租居多" # 网点还车 if float(row["还车次数"])/row["FF"]>0.5: data_total["还车偏好"]="非网点还车" else: data_total["还车偏好"]="网点还车" # 月均订单 if row["FF"]/row["月数"]>6: data_total["月均单量"]="月均单量大于6" else: data_total["月均单量"]="月均单量小于6"data_total
| 用户ID | F | M | R | R1 | RR | FF | MM | score | level | order_num | 月数 | 自取次数 | 优惠券使用次数 | 还车次数 | 取车偏好 | 还车偏好 | 月均单量 |
---|
0 | 1 | 32 | 3517.0 | 2021/01/25 | -330 | 0.980519 | 0.673913 | 0.663953 | 71.392801 | 一等 | 32 | 32 | 11 | 23 | 13 | 时租居多 | 网点还车 | 月均单量小于6 |
---|
1 | 10 | 29 | 2600.0 | 2021/01/23 | -328 | 0.977922 | 0.608696 | 0.490345 | 59.306896 | 一等 | 29 | 29 | 16 | 24 | 12 | 时租居多 | 网点还车 | 月均单量小于6 |
---|
2 | 100 | 31 | 3906.0 | 2021/01/11 | -316 | 0.962338 | 0.652174 | 0.737599 | 74.995376 | 一等 | 31 | 31 | 12 | 21 | 7 | 时租居多 | 网点还车 | 月均单量小于6 |
---|
3 | 1000 | 24 | 2255.0 | 2021/01/07 | -312 | 0.957143 | 0.500000 | 0.425028 | 52.358847 | 二等 | 24 | 24 | 13 | 15 | 11 | 时租居多 | 网点还车 | 月均单量小于6 |
---|
4 | 10009 | 1 | 71.0 | 2019/04/09 | 327 | 0.127273 | 0.000000 | 0.011549 | 2.602010 | 四等 | 1 | 1 | 1 | 0 | 1 | 时租居多 | 网点还车 | 月均单量小于6 |
---|
... | ... | ... | ... | ... | ... | ... | ... | ... | ... | ... | ... | ... | ... | ... | ... | ... | ... | ... |
---|
5924 | 9977 | 1 | 19.0 | 2020/10/27 | -240 | 0.863636 | 0.000000 | 0.001704 | 13.056779 | 三等 | 1 | 1 | 0 | 1 | 0 | 时租居多 | 网点还车 | 月均单量小于6 |
---|
5925 | 998 | 34 | 3634.0 | 2021/02/06 | -342 | 0.996104 | 0.717391 | 0.686104 | 74.042566 | 一等 | 34 | 34 | 18 | 24 | 13 | 时租居多 | 网点还车 | 月均单量小于6 |
---|
5926 | 999 | 33 | 3356.0 | 2021/01/27 | -332 | 0.983117 | 0.695652 | 0.633472 | 70.146388 | 一等 | 33 | 33 | 12 | 19 | 10 | 时租居多 | 网点还车 | 月均单量小于6 |
---|
5927 | 9993 | 1 | 125.0 | 2020/11/04 | -248 | 0.874026 | 0.000000 | 0.021772 | 14.416713 | 三等 | 1 | 1 | 0 | 1 | 1 | 时租居多 | 网点还车 | 月均单量小于6 |
---|
5928 | 9994 | 1 | 135.0 | 2020/08/22 | -174 | 0.777922 | 0.000000 | 0.023665 | 13.088748 | 三等 | 1 | 1 | 1 | 0 | 0 | 时租居多 | 网点还车 | 月均单量小于6 |
---|
5929 rows × 18 columns
用户生命周期
def user_life(data,a,b,c): List_1=[] for index,row in data.iterrows():# print(row[a])# print(index) if row[a]==1: row[c]="引入期" elif row[a]>1 and row[a]<3 and row[b]<=200: row[c]="成长期" elif row[a]>=3 and row[b]<=300: row[c]="休眠期" elif row[b]>300: row[c]="流失期" else: row[c]="其他"# print(row[c]) List_1.append(row) data_1=pd.DataFrame(List_1)# print(data_1) return data_1Userlife=pd.DataFrame()Userlife=user_life(data_total,"F","R1","生命周期")Userlife.head(10)
| 用户ID | F | M | R | R1 | RR | FF | MM | score | level | order_num | 月数 | 自取次数 | 优惠券使用次数 | 还车次数 | 取车偏好 | 还车偏好 | 月均单量 | 生命周期 |
---|
0 | 1 | 32 | 3517.0 | 2021/01/25 | -330 | 0.980519 | 0.673913 | 0.663953 | 71.392801 | 一等 | 32 | 32 | 11 | 23 | 13 | 时租居多 | 网点还车 | 月均单量小于6 | 休眠期 |
---|
1 | 10 | 29 | 2600.0 | 2021/01/23 | -328 | 0.977922 | 0.608696 | 0.490345 | 59.306896 | 一等 | 29 | 29 | 16 | 24 | 12 | 时租居多 | 网点还车 | 月均单量小于6 | 休眠期 |
---|
2 | 100 | 31 | 3906.0 | 2021/01/11 | -316 | 0.962338 | 0.652174 | 0.737599 | 74.995376 | 一等 | 31 | 31 | 12 | 21 | 7 | 时租居多 | 网点还车 | 月均单量小于6 | 休眠期 |
---|
3 | 1000 | 24 | 2255.0 | 2021/01/07 | -312 | 0.957143 | 0.500000 | 0.425028 | 52.358847 | 二等 | 24 | 24 | 13 | 15 | 11 | 时租居多 | 网点还车 | 月均单量小于6 | 休眠期 |
---|
4 | 10009 | 1 | 71.0 | 2019/04/09 | 327 | 0.127273 | 0.000000 | 0.011549 | 2.602010 | 四等 | 1 | 1 | 1 | 0 | 1 | 时租居多 | 网点还车 | 月均单量小于6 | 引入期 |
---|
5 | 1001 | 28 | 3074.0 | 2021/01/24 | -329 | 0.979221 | 0.586957 | 0.580083 | 64.167223 | 一等 | 28 | 28 | 12 | 18 | 12 | 时租居多 | 网点还车 | 月均单量小于6 | 休眠期 |
---|
6 | 10014 | 1 | 49.0 | 2020/08/25 | -177 | 0.781818 | 0.000000 | 0.007384 | 12.170287 | 三等 | 1 | 1 | 1 | 1 | 0 | 时租居多 | 网点还车 | 月均单量小于6 | 引入期 |
---|
7 | 10016 | 1 | 67.0 | 2020/07/19 | -140 | 0.733766 | 0.000000 | 0.010791 | 11.653976 | 三等 | 1 | 1 | 0 | 1 | 0 | 时租居多 | 网点还车 | 月均单量小于6 | 引入期 |
---|
8 | 1002 | 29 | 3316.0 | 2021/01/03 | -308 | 0.951948 | 0.608696 | 0.625899 | 67.050569 | 一等 | 29 | 29 | 12 | 19 | 15 | 时租居多 | 网点还车 | 月均单量小于6 | 休眠期 |
---|
9 | 10023 | 1 | 81.0 | 2020/09/09 | -192 | 0.801299 | 0.000000 | 0.013442 | 12.825993 | 三等 | 1 | 1 | 1 | 1 | 0 | 时租居多 | 网点还车 | 月均单量小于6 | 引入期 |
---|
"休眠期" in Userlife["生命周期"].values
True
zq=Userlife.groupby("生命周期")yr=zq.get_group("引入期")["生命周期"].count()cz=zq.get_group("成长期")["生命周期"].count()xm=zq.get_group("休眠期")["生命周期"].count()ls=zq.get_group("流失期")["生命周期"].count()x=["引入期","成长期","休眠期","流失期"]y=[yr,cz,xm,ls]for i in range(len(x)): print(x[i],y[i])my_Font = Font_manager.FontPropertIEs(fname="C:/Users/wty_pc/Anaconda3/lib/site-packages/matplotlib/mpl-data/Fonts/ttf/STSONG.TTF",size = 18)plt.figure(figsize=(25,10),dpi=80)plt.rcParams['Font.sans-serif']=['STSONG'] #中文标签设置# plt.rcParams['axes.unicode_minus']=False #用来正常显示坐标负号rects=plt.bar(x,y,0.3,color=["r","g","b","y"])plt.xticks(x,x,FontpropertIEs=my_Font)for rect in rects: height=rect.get_height() plt.text(rect.get_x()+rect.get_wIDth()/2,height+1,str(height),Fontsize=18,ha="center")plt.show()
引入期 2528成长期 895休眠期 2414流失期 32
用户评分统计
# 评分 #0分代表未参与评分,可以统计用户的参与度sql='''select date_format(付款时间,"%Y/%m") 付款年月,评分,count(distinct 用户ID) as user_num,count(*) as order_num from paper_data group by date_format(付款时间,"%Y/%m"),评分;'''pinfen_num=get_MysqL_data(DB,sql)pinfen_num["评分"]=pinfen_num["评分"].astype(int)pinfen_num["参与度"]=pinfen_num["user_num"]/pinfen_num["order_num"]pinfen_num.dtypes
付款年月 object评分 int32user_num int64order_num int64参与度 float64dtype: object
# 用户评分参与度=有评分的用户/下单用户量import numpy as np# 按评分分组pf_group=pinfen_num.groupby(["评分"])# ID_name# 取出分组的值# 方法一List_pf=[]for key,value in pf_group: List_pf.append(value.reset_index(drop="true"))# 方法二格式化成列表或者字典,详见后面一个代码块x=List_pf[0]["付款年月"]y=[]for i in range(len(List_pf)): y.append(List_pf[i]["参与度"])# print("x:",x.values)# print("y:",y[1])my_Font = Font_manager.FontPropertIEs(fname="C:/Users/wty_pc/Anaconda3/lib/site-packages/matplotlib/mpl-data/Fonts/ttf/STSONG.TTF",size = 15)fig=plt.figure(figsize=(20,40),dpi=80)colors=["r","g","b"]for i in range(1,12): ax=fig.add_subplot(11,1,i) ax.plot(x,y[i-1],color=colors[np.random.randint(3)],label="{}分".format(i-1)) x_label="评分为{}的趋势".format(i-1) ax.set_xlabel(x_label,FontpropertIEs=my_Font) ax.set_ylabel("参与度",FontpropertIEs=my_Font) ax.legend() #调整子图间距 plt.tight_layout()plt.show()
groupby取数的技巧
# groupby 格式化成列表 为转换成字典做铺垫a=List(pinfen_num.groupby(["评分"]))# 列表的每个元素是个元组,每个元组的值为分组键和值(datafarme)a[0][1].reset_index(drop = True)# print(type(a[1]))
| 付款年月 | 评分 | user_num | order_num | 参与度 |
---|
0 | 2019/01 | 0 | 230 | 241 | 0.954357 |
---|
1 | 2019/02 | 0 | 206 | 213 | 0.967136 |
---|
2 | 2019/03 | 0 | 239 | 258 | 0.926357 |
---|
3 | 2019/04 | 0 | 209 | 216 | 0.967593 |
---|
4 | 2019/05 | 0 | 213 | 223 | 0.955157 |
---|
5 | 2019/06 | 0 | 217 | 230 | 0.943478 |
---|
6 | 2019/07 | 0 | 229 | 239 | 0.958159 |
---|
7 | 2019/08 | 0 | 216 | 222 | 0.972973 |
---|
8 | 2019/09 | 0 | 214 | 229 | 0.934498 |
---|
9 | 2019/10 | 0 | 232 | 244 | 0.950820 |
---|
10 | 2019/11 | 0 | 225 | 235 | 0.957447 |
---|
11 | 2019/12 | 0 | 239 | 247 | 0.967611 |
---|
12 | 2020/01 | 0 | 271 | 287 | 0.944251 |
---|
13 | 2020/02 | 0 | 187 | 200 | 0.935000 |
---|
14 | 2020/03 | 0 | 221 | 230 | 0.960870 |
---|
15 | 2020/04 | 0 | 216 | 230 | 0.939130 |
---|
16 | 2020/05 | 0 | 219 | 227 | 0.964758 |
---|
17 | 2020/06 | 0 | 207 | 218 | 0.949541 |
---|
18 | 2020/07 | 0 | 224 | 232 | 0.965517 |
---|
19 | 2020/08 | 0 | 239 | 250 | 0.956000 |
---|
20 | 2020/09 | 0 | 224 | 233 | 0.961373 |
---|
21 | 2020/10 | 0 | 240 | 250 | 0.960000 |
---|
22 | 2020/11 | 0 | 220 | 227 | 0.969163 |
---|
23 | 2020/12 | 0 | 218 | 230 | 0.947826 |
---|
24 | 2021/01 | 0 | 238 | 251 | 0.948207 |
---|
25 | 2021/02 | 0 | 56 | 57 | 0.982456 |
---|
# groupby 格式化成字典a=dict(List(pinfen_num.groupby(["评分"])))# type(a[0])a[0].reset_index(drop=True)
| 付款年月 | 评分 | user_num | order_num | 参与度 |
---|
0 | 2019/01 | 0 | 230 | 241 | 0.954357 |
---|
1 | 2019/02 | 0 | 206 | 213 | 0.967136 |
---|
2 | 2019/03 | 0 | 239 | 258 | 0.926357 |
---|
3 | 2019/04 | 0 | 209 | 216 | 0.967593 |
---|
4 | 2019/05 | 0 | 213 | 223 | 0.955157 |
---|
5 | 2019/06 | 0 | 217 | 230 | 0.943478 |
---|
6 | 2019/07 | 0 | 229 | 239 | 0.958159 |
---|
7 | 2019/08 | 0 | 216 | 222 | 0.972973 |
---|
8 | 2019/09 | 0 | 214 | 229 | 0.934498 |
---|
9 | 2019/10 | 0 | 232 | 244 | 0.950820 |
---|
10 | 2019/11 | 0 | 225 | 235 | 0.957447 |
---|
11 | 2019/12 | 0 | 239 | 247 | 0.967611 |
---|
12 | 2020/01 | 0 | 271 | 287 | 0.944251 |
---|
13 | 2020/02 | 0 | 187 | 200 | 0.935000 |
---|
14 | 2020/03 | 0 | 221 | 230 | 0.960870 |
---|
15 | 2020/04 | 0 | 216 | 230 | 0.939130 |
---|
16 | 2020/05 | 0 | 219 | 227 | 0.964758 |
---|
17 | 2020/06 | 0 | 207 | 218 | 0.949541 |
---|
18 | 2020/07 | 0 | 224 | 232 | 0.965517 |
---|
19 | 2020/08 | 0 | 239 | 250 | 0.956000 |
---|
20 | 2020/09 | 0 | 224 | 233 | 0.961373 |
---|
21 | 2020/10 | 0 | 240 | 250 | 0.960000 |
---|
22 | 2020/11 | 0 | 220 | 227 | 0.969163 |
---|
23 | 2020/12 | 0 | 218 | 230 | 0.947826 |
---|
24 | 2021/01 | 0 | 238 | 251 | 0.948207 |
---|
25 | 2021/02 | 0 | 56 | 57 | 0.982456 |
---|
pinfen_num.groupby(["评分"]).size()
评分0 261 262 263 264 265 266 267 268 269 2610 26dtype: int64
pinfen_num.groupby(["评分"]).count()
| 付款年月 | user_num | order_num | 参与度 |
---|
评分 | | | | |
---|
0 | 26 | 26 | 26 | 26 |
---|
1 | 26 | 26 | 26 | 26 |
---|
2 | 26 | 26 | 26 | 26 |
---|
3 | 26 | 26 | 26 | 26 |
---|
4 | 26 | 26 | 26 | 26 |
---|
5 | 26 | 26 | 26 | 26 |
---|
6 | 26 | 26 | 26 | 26 |
---|
7 | 26 | 26 | 26 | 26 |
---|
8 | 26 | 26 | 26 | 26 |
---|
9 | 26 | 26 | 26 | 26 |
---|
10 | 26 | 26 | 26 | 26 |
---|
# 每个评分最小参与度pinfen_num["参与度"].groupby(pinfen_num["评分"]).min()pinfen_num.groupby(pinfen_num["评分"]).min()["参与度"]pinfen_num.groupby(pinfen_num["评分"])["参与度"].min()
评分0 0.9263571 0.9236952 0.9177493 0.9256204 0.9120005 0.9346946 0.9163187 0.9299618 0.9295779 0.94009210 0.928571name: 参与度, dtype: float64
# 按评分选取多列# pinfen_num.groupby(pinfen_num["评分"])[["参与度","order_num"]].min()pinfen_num[["参与度","order_num"]].groupby(pinfen_num["评分"]).min()
| 参与度 | order_num |
---|
评分 | | |
---|
0 | 0.926357 | 57 |
---|
1 | 0.923695 | 47 |
---|
2 | 0.917749 | 67 |
---|
3 | 0.925620 | 61 |
---|
4 | 0.912000 | 62 |
---|
5 | 0.934694 | 68 |
---|
6 | 0.916318 | 52 |
---|
7 | 0.929961 | 62 |
---|
8 | 0.929577 | 65 |
---|
9 | 0.940092 | 48 |
---|
10 | 0.928571 | 66 |
---|
# 多维分组用unstack可以解成二维表(不堆叠)a=pinfen_num["参与度"].groupby([pinfen_num["付款年月"],pinfen_num["评分"]]).min().unstack()a# a.shape
评分 | 0 | 1 | 2 | 3 | 4 | 5 | 6 | 7 | 8 | 9 | 10 |
---|
付款年月 | | | | | | | | | | | |
---|
2019/01 | 0.954357 | 0.950000 | 0.971074 | 0.966805 | 0.967871 | 0.976378 | 0.937500 | 0.961538 | 0.935743 | 0.950617 | 0.957806 |
---|
2019/02 | 0.967136 | 0.938967 | 0.972477 | 0.952000 | 0.946341 | 0.943925 | 0.931373 | 0.944444 | 0.934579 | 0.954545 | 0.944954 |
---|
2019/03 | 0.926357 | 0.945148 | 0.928000 | 0.958716 | 0.939759 | 0.940928 | 0.966805 | 0.930612 | 0.956349 | 0.965368 | 0.937799 |
---|
2019/04 | 0.967593 | 0.966825 | 0.970443 | 0.946903 | 0.925581 | 0.978632 | 0.990741 | 0.949580 | 0.977679 | 0.969957 | 0.944700 |
---|
2019/05 | 0.955157 | 0.953975 | 0.946903 | 0.947154 | 0.974576 | 0.952174 | 0.957627 | 0.949153 | 0.962617 | 0.944206 | 0.970588 |
---|
2019/06 | 0.943478 | 0.923695 | 0.948598 | 0.936937 | 0.948819 | 0.958159 | 0.957031 | 0.965217 | 0.953390 | 0.951327 | 0.945378 |
---|
2019/07 | 0.958159 | 0.950413 | 0.968889 | 0.942222 | 0.950000 | 0.954167 | 0.957806 | 0.935849 | 0.948339 | 0.957983 | 0.977876 |
---|
2019/08 | 0.972973 | 0.937500 | 0.945148 | 0.956897 | 0.973684 | 0.968037 | 0.921569 | 0.970711 | 0.961702 | 0.948936 | 0.958506 |
---|
2019/09 | 0.934498 | 0.977876 | 0.948837 | 0.959276 | 0.972851 | 0.973913 | 0.970732 | 0.950893 | 0.954751 | 0.946565 | 0.970588 |
---|
2019/10 | 0.950820 | 0.969957 | 0.982456 | 0.951965 | 0.927419 | 0.944882 | 0.954128 | 0.960526 | 0.946429 | 0.950207 | 0.967611 |
---|
2019/11 | 0.957447 | 0.949580 | 0.982759 | 0.960870 | 0.942308 | 0.965517 | 0.965517 | 0.960159 | 0.934694 | 0.964467 | 0.965217 |
---|
2019/12 | 0.967611 | 0.940639 | 0.948936 | 0.937238 | 0.967078 | 0.940594 | 0.958678 | 0.929961 | 0.946667 | 0.961702 | 0.930736 |
---|
2020/01 | 0.944251 | 0.945607 | 0.961864 | 0.977876 | 0.943478 | 0.976959 | 0.941909 | 0.965517 | 0.951020 | 0.948207 | 0.928571 |
---|
2020/02 | 0.935000 | 0.967890 | 0.963134 | 0.963115 | 0.973333 | 0.944444 | 0.972973 | 0.934783 | 0.969432 | 0.948498 | 0.967136 |
---|
2020/03 | 0.960870 | 0.985915 | 0.941423 | 0.943478 | 0.941441 | 0.977578 | 0.973913 | 0.963636 | 0.946860 | 0.964844 | 0.955157 |
---|
2020/04 | 0.939130 | 0.927203 | 0.938224 | 0.931452 | 0.952174 | 0.945946 | 0.961702 | 0.959184 | 0.945525 | 0.940092 | 0.942387 |
---|
2020/05 | 0.964758 | 0.935484 | 0.917749 | 0.944664 | 0.978070 | 0.959276 | 0.933333 | 0.963855 | 0.954717 | 0.963563 | 0.974684 |
---|
2020/06 | 0.949541 | 0.959091 | 0.952790 | 0.925620 | 0.951542 | 0.963855 | 0.953052 | 0.964126 | 0.958159 | 0.962617 | 0.941909 |
---|
2020/07 | 0.965517 | 0.966245 | 0.949219 | 0.951754 | 0.912000 | 0.945701 | 0.924528 | 0.955645 | 0.967442 | 0.942748 | 0.942652 |
---|
2020/08 | 0.956000 | 0.939759 | 0.926087 | 0.990610 | 0.931034 | 0.944206 | 0.916318 | 0.954733 | 0.976378 | 0.961373 | 0.966038 |
---|
2020/09 | 0.961373 | 0.960000 | 0.958904 | 0.956332 | 0.944915 | 0.980952 | 0.935345 | 0.937238 | 0.960000 | 0.955357 | 0.945607 |
---|
2020/10 | 0.960000 | 0.935345 | 0.943231 | 0.952381 | 0.926740 | 0.941704 | 0.964912 | 0.936000 | 0.931452 | 0.954751 | 0.956140 |
---|
2020/11 | 0.969163 | 0.968872 | 0.952381 | 0.946667 | 0.918919 | 0.960159 | 0.942222 | 0.964758 | 0.929577 | 0.955157 | 0.947581 |
---|
2020/12 | 0.947826 | 0.951542 | 0.957983 | 0.946667 | 0.967593 | 0.951754 | 0.948113 | 0.958175 | 0.951754 | 0.948718 | 0.959016 |
---|
2021/01 | 0.948207 | 0.936364 | 0.961207 | 0.931727 | 0.950192 | 0.934694 | 0.967078 | 0.956693 | 0.961089 | 0.957447 | 0.944444 |
---|
2021/02 | 0.982456 | 0.978723 | 0.970149 | 0.983607 | 1.000000 | 0.970588 | 1.000000 | 1.000000 | 0.969231 | 1.000000 | 0.984848 |
---|
#多维分组堆叠成多索引 b=pinfen_num["参与度"].groupby([pinfen_num["付款年月"],pinfen_num["评分"]]).min()b# b.shape
付款年月 评分2019/01 0 0.954357 1 0.950000 2 0.971074 3 0.966805 4 0.967871 ... 2021/02 6 1.000000 7 1.000000 8 0.969231 9 1.000000 10 0.984848name: 参与度, Length: 286, dtype: float64
pinfen_num["参与度"].groupby(pinfen_num["评分"]).describe().unstack()
评分count 0 26.000000 1 26.000000 2 26.000000 3 26.000000 4 26.000000 ... max 6 1.000000 7 1.000000 8 0.977679 9 1.000000 10 0.984848Length: 88, dtype: float64
pinfen_num["参与度"].groupby(pinfen_num["评分"]).describe()
| count | mean | std | min | 25% | 50% | 75% | max |
---|
评分 | | | | | | | | |
---|
0 | 26.0 | 0.955372 | 0.013188 | 0.926357 | 0.947921 | 0.956723 | 0.965327 | 0.982456 |
---|
1 | 26.0 | 0.952408 | 0.016614 | 0.923695 | 0.939165 | 0.950207 | 0.966680 | 0.985915 |
---|
2 | 26.0 | 0.954187 | 0.016478 | 0.917749 | 0.945586 | 0.952585 | 0.967450 | 0.982759 |
---|
3 | 26.0 | 0.952420 | 0.015517 | 0.925620 | 0.943775 | 0.951860 | 0.959136 | 0.990610 |
---|
4 | 26.0 | 0.951066 | 0.021123 | 0.912000 | 0.940180 | 0.949409 | 0.967802 | 1.000000 |
---|
5 | 26.0 | 0.957505 | 0.014377 | 0.934694 | 0.944554 | 0.956163 | 0.969950 | 0.980952 |
---|
6 | 26.0 | 0.954035 | 0.020423 | 0.916318 | 0.938602 | 0.957329 | 0.966483 | 1.000000 |
---|
7 | 26.0 | 0.954730 | 0.015248 | 0.929961 | 0.945621 | 0.957434 | 0.963801 | 1.000000 |
---|
8 | 26.0 | 0.953291 | 0.013283 | 0.929577 | 0.946488 | 0.954053 | 0.961549 | 0.977679 |
---|
9 | 26.0 | 0.956510 | 0.011825 | 0.940092 | 0.948773 | 0.954954 | 0.962388 | 1.000000 |
---|
10 | 26.0 | 0.954920 | 0.014803 | 0.928571 | 0.944508 | 0.955649 | 0.966862 | 0.984848 |
---|
总结
以上是内存溢出为你收集整理的python连接Mysql共享汽车行业案例分析全部内容,希望文章能够帮你解决python连接Mysql共享汽车行业案例分析所遇到的程序开发问题。
如果觉得内存溢出网站内容还不错,欢迎将内存溢出网站推荐给程序员好友。
评论列表(0条)