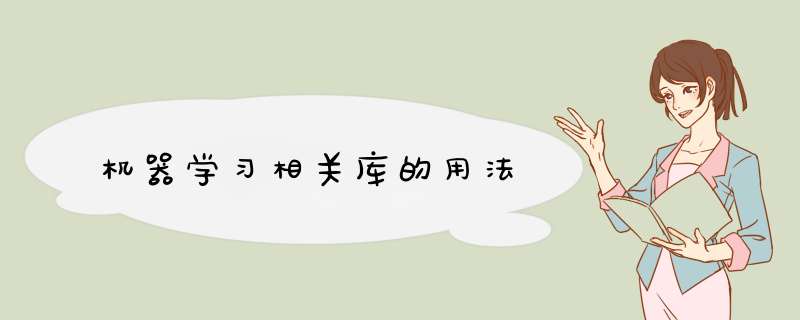
机器学习相关库的用法
机器学习相关库和函数
来自:https://zhuanlan.zhihu.com/p/342941676
1、数据预处理(preprocessing)
相关库和函数 | 作用 |
---|
from sklearn.preprocessing import StandardScaler数据标准化
from sklearn.preprocessing import MinMaxScaler数据缩放到[0,1]间
from sklearn.preprocessing import Normalizer数据归一化:单位化向量
from sklearn.preprocessing import OneHotEncoder数据进行onehot编码
from sklearn.preprocessing import Binarizer对定量变量二值化:设定一个阈值,大于阈值的赋值为1,小于等于阈值的赋值为0
from sklearn.preprocessing import OrdinaryEncoder数值编码:将字符串文字编码为1、2、3、4…
pd.fillna(填充值)缺失值填充
pd.quantile(某个分位数)获取分位数值
pd.mask(condition,other=None)当condition为true时,用other来进行替换
pd.where(condition,other=None)当condition为false时,用other来进行替换
2、
特征选择(feature_selection)
2.1、过滤式特征
选择
相关库 | 特征变量类型 | 目标变量类型 |
---|
from sklearn.feature_selection import VarianceThreshold连续型均可
from sklearn.feature_selection import chi2(卡方检验)分类型分类型
from sklearn.feature_selection import f_classif(F检验:方差分析)连续型分类型
from sklearn.feature_selection import mutual_info_classif(互信息分类)连续型分类型
from sklearn.feature_selection import f_regression(相关系数)连续型连续型
from sklearn.feature_selection import mutual_info_regression(互信息回归)连续型连续型
相关统计变量解释:
互信息:度量 X 和 Y 共享的信息,它度量知道这两个变量其中一个,对另一个不确定度减少的程度。值越大越相关 ,0表示特征和标签完全独立。
# SelectKBest与表格中的系数组合使用,最终选取出特定数量的特征
from sklearn.feature_selection import SelectKBest
from sklearn.feature_selection import chi2
#选择K个最好的特征,返回选择特征后的数据,输出为选择后的特征矩阵
SelectKBest(chi2, k=2).fit_transform(iris.data, iris.target)
2.2、包裹式特征选择
from sklearn.feature_selection import RFE
from sklearn.linear_model import LogisticRegression
#递归特征消除法,返回特征选择后的数据
#参数estimator为基模型
#参数n_features_to_select为选择的特征个数
RFE(estimator=LogisticRegression(), n_features_to_select=2).fit_transform(iris.data, iris.target)
2.3、嵌入式特征选择
2.3.1 基于惩罚项的特征选择
from sklearn.feature_selection import SelectFromModel
from sklearn.linear_model import LogisticRegression
#带L1惩罚项的逻辑回归作为基模型的特征选择
SelectFromModel(LogisticRegression(penalty="l1", C=0.1)).fit_transform(iris.data, iris.target)
2.3.2 基于树
模型的特征选择
from sklearn.feature_selection import SelectFromModel
from sklearn.ensemble import GradientBoostingClassifier
#GBDT作为基模型的特征选择
SelectFromModel(GradientBoostingClassifier()).fit_transform(iris.data, iris.target)
SelectFromModel使用说明:https://blog.csdn.net/weixin_46072771/article/details/106190351
模型方法 | 作用 |
---|
SelectFromModel.fit(X,y)根据训练集训练模型,返回是模型,可以继续调用selector.estimator_.coef_或者selector.estimator_.feature_importances_获取特征重要性并确定特征选择阈值
SelectFromModel.transform(X,y)将X缩小为选定的特征,返回值为去除不相关特征后的数据,一般是调用fit()方法之后再调用
SelectFromModel.fit_transform(X,y)根据训练集训练并返回去除不相关特征后的数据,一步到位
3、降维
当特征选择完成后,可以直接训练模型了,但是可能由于特征矩阵过大,导致计算量大,训练时间长的问题,因此降低特征矩阵维度也是必不可少的。常见的降维方法除了以上提到的基于L1惩罚项的模型以外,另外还有主成分分析法(PCA)和线性判别分析(LDA),线性判别分析本身也是一个分类模型。PCA和LDA有很多的相似点,其本质是要将原始的样本映射到维度更低的样本空间中,但是PCA和LDA的映射目标不一样:PCA是为了让映射后的样本具有最大的发散性;而LDA是为了让映射后的样本有最好的分类性能。所以说PCA是一种无监督的降维方法,而LDA是一种有监督的降维方法。
相关库 | 作用 |
---|
from sklearn.decomposition import PCA主成分降维
from sklearn.lda import LDALDA降维
4、模型选择(model_selection)
模型选择是机器学习中的重要环节,涉及到的 *** 作包括数据集切分、参数调整和验证等。
相关库 | 作用 |
---|
from model_selection import train_test_split切分数据集和测试集,可设置切分比例
from model_selection import cross_val_score交叉验证,默认K=5折,最后返回K个评分
from model_selection import GridSearchCV调参常用方法,通过字典类型设置一组候选参数,并制定度量标准,最后返回评分最高的参数
5、指标度量(metrics)
评论列表(0条)